How to create a semantic core for your app?
The semantic core of an app is the foundation, the basis for working with the optimization of mobile apps. Almost any topic that reveals the essence of ASO optimization will concern the semantic core. In our guide for beginner ASO specialists, we will touch on the sources of semantics, how to collect and analyze search queries, and what to do with them next.
Semantic core - sources of keywords
Summer is just around the corner, so no one will be surprised by the interest in being fit, so we are on trend. This is how a search query is born - out of need. Almost all keywords and phrases are reflections of user needs. Anything that in some way solves a problem satisfies a need, or helps users ends up in the search query field. Please note that we do not go to the App Store with informational questions like who killed Kennedy or why dinosaurs became extinct
Only with a task or need - online delivery, meditation and sounds of nature, habit tracker.
The first short conclusion is that queries for the semantic core are functional, not informational.
Therefore, in solving our task of getting our body ready for the beach season, we can already formulate a number of search queries in this regard:
- fitness at home
- weight loss
- lose weight
- home fitness
Each of these search queries will have its own search results, but if you check, almost all of them will show a more or less uniform Top Application. Let's check:
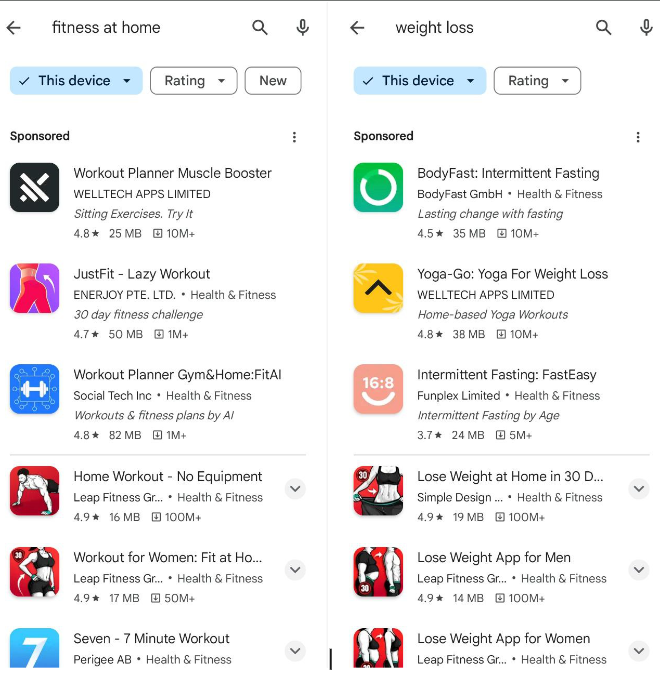
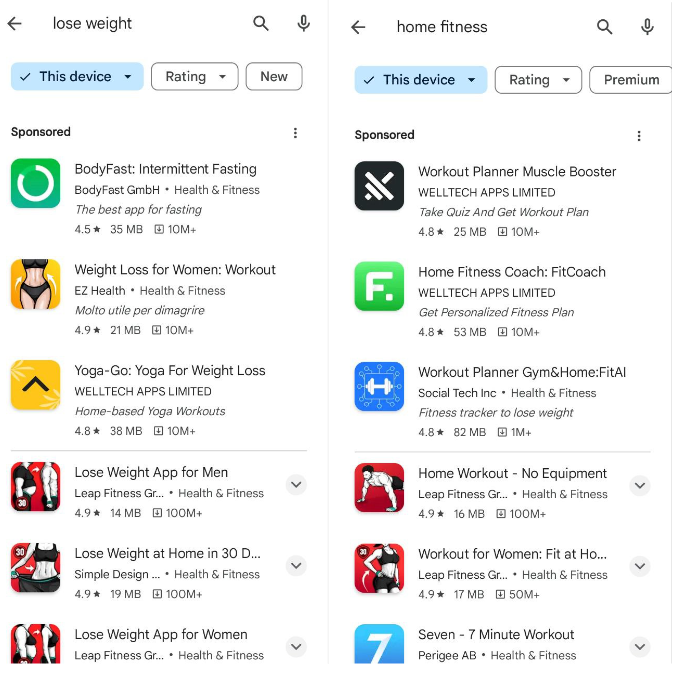
It’s worth mentioning right away that the first three places in the search results are advertising (there’s even a special icon there). And the user, without additional scrolling, only sees the first two places in the search.
The second brief conclusion is that places in the Top are very important, installations will most often occur from the top 10 apps in the search results, although of course no one limits scrolling further. This is what motivates you to optimize your app, when without using advertising, meeting with users is still possible.
The second conclusion is that the sources of semantics are the needs of the user; they are also the functionality of apps.
Now, let's go back to the app store. Just now, when entering a search query, we most likely used the auto-suggestion - right?
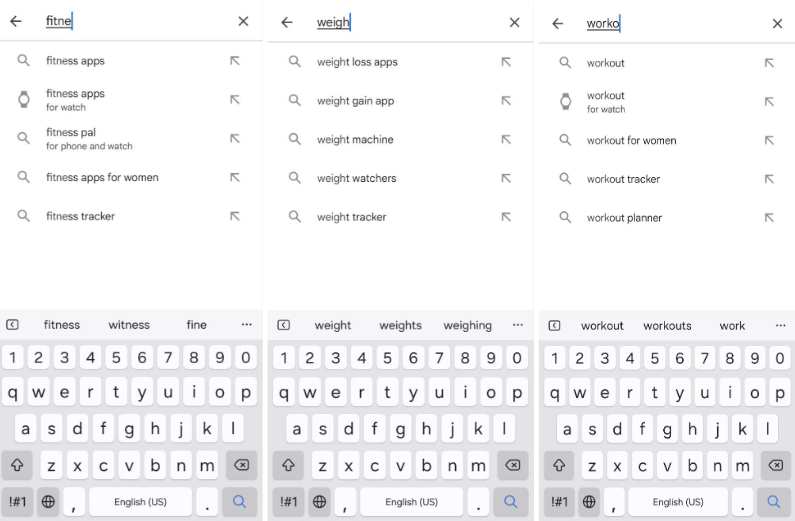
This is what the app store suggestion looks like - this is an already existing history of requests of this type among users. Thus, the store suggests and saves our time, and also works as a clarification - we are interested in fitness, but what kind? This way we can choose a more accurate match to our expectations - fitness for home, fitness tracker, fitness for weight loss. But if our task is to collect as many queries as possible that suit us, then hints are a valuable source. So out of four requests that you just came up with we receive seven or eight tips from the store and voila - our list already looks like a list.
The third conclusion - store suggestions are a valuable source of semantics.
What else can we pay our attention to when collecting our keywords? To competitors. Those same apps that ended up in search results for queries that interest us (without departing from the example, let there be a fitness app). We can visit the page of each of them and familiarize ourselves with the information that is posted there. For now, without delving into the jungle of text optimization, we are skimming the surface of search results.
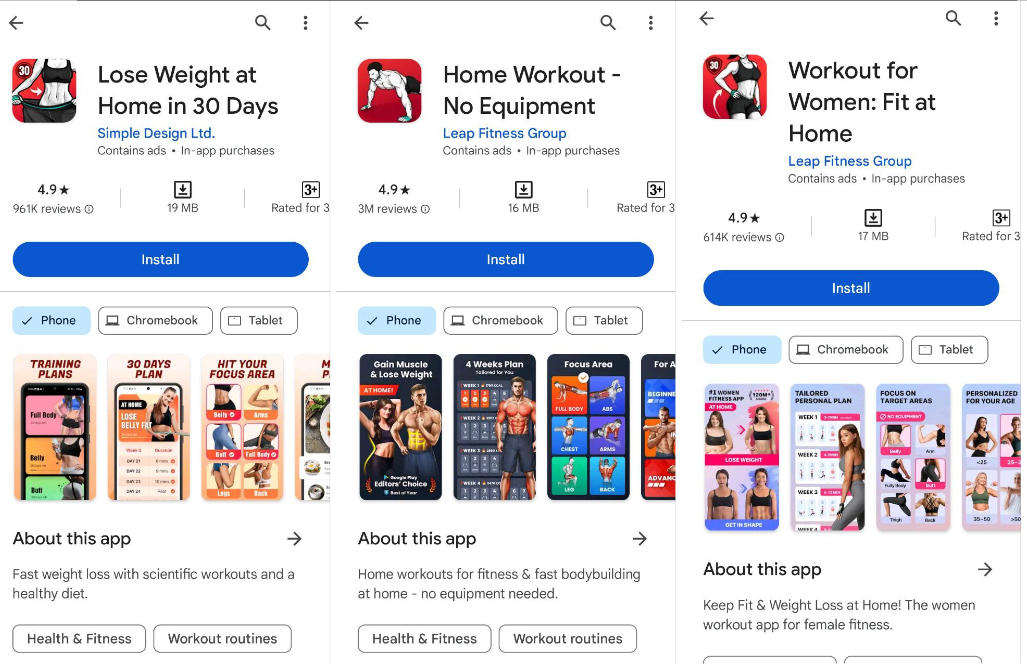
Looking at the title and the beginning of the description of apps in the Top search results, we add new key relevant phrases to our list - “fitness for women”, “lose weight in 30 days”, “workouts at home”, “home workouts”, “healthy eating”.
So, without a game-changing solution in the form of analytical tools, we have exhausted our ability to collect search queries, but the main message is to understand the relationship between what the user is looking for, how key queries are formed and what is reflected in the search results. We managed this.
A short conclusion - the user's needs that brought him to the app store will form the basis of the semantic core of the relevant application. All that remains is to clarify a few terms:
- Relevance is the correspondence of all three signs, a need from the user - a request to the app store - a suggestion and a key phrase.
- keywords, search phrases, keys - these are all absolutely identical concepts that represent a verbal expression of the user’s needs.
- search results are a list of apps or games that the app store generates for each request.
- when an app is shown in the list for a keyword, this is called indexing or that the app is indexed for that search query.
I think it has already become quite clear that user requests correlate with those phrases and words that are on the app page. They are contained in their title, description and subtitle - what is generally called app metadata. It is for them that we will form our semantic core.
Our main task, in addition to the list of keys, is to make it clear to the app store what user search queries we need to be shown for. All this happens based on metadata and takes into account the app store - where we are located. We promise that at the very end everything will become crystal clear, but for now let’s return to the roots, namely the semantic core.
The essence of an app semantic core
The main purpose of creating a list of keywords, also known as a semantic core, is to compare user queries in all their diversity and app functionality. This means that our task is not only to find and collect a list of keywords, but also to filter them according to two criteria:
- according to the requests made by users on the store.
- according to the relevance of the app or game.
A simple example to dot the i's and cross the t's:
The interval timer app is a set of all kinds of timers for functional training, from a trivial stopwatch to customizable time intervals. All search queries “training timer”, “wod timer”, “crossfit timer” are suitable and form the basis of the semantic core. But “kitchen timer” and “cooking timer”, which are very similar in meaning, are not relevant at all. Of course, we can argue with this, and you can boil eggs using a training timer, but we are unlikely to be able to convince users of this. We check the relevance of keywords by the simplest and most transparent correspondence between their app functions, on the one hand, and user tasks, on the other hand.
Further, it is worth noting that search phrases can actually be phrases (multiple words, prepositions and conjunctions), or they can be one or two words. Thanks to auto suggestions, the user does not need to type a lot, but simply select from the list. This is why in the era of brevity and abbreviations, we still see long search phrases (but usually no more than 4 words).
We can begin the formation of semantics, as in this guide, with the logic and meaning of the app. Then, we will follow the store's tips - we will look there not only at the list of possible participants in our core, but also at the wording themselves. How, what word order, declensions and forms are used by our potential users. And here, in fact, our possibilities are exhausted, and the list of words can turn out to be quite short. The next point in our guide will allow us to significantly expand it and make it as informative as possible.
The semantic core of the app is a list of relevant search queries. However, in addition to the sign “suits the functionality of the app,” we come to a very important indicator of the keyword - the popularity of the search query. How many users are potentially interested in a particular request? Here, it’s time to mention not only the qualitative characteristics of the keyword but also the quantitative ones. Traffic is one of the most important indicators of keywords. It is this indicator that shows us how many users per day searched for a particular request in the application store. And here, we can no longer do without special analytical tools. We will go through the analytics capabilities in detail step by step and assemble our semantic core. For now, let's summarize the keywords:
By meaning:
- relevant
- irrelevant
By length:
- short tail - search queries of two or one-word
- long-tail - key phrases of several words
By traffic:
- high frequency
- mid-frequency
- low frequency
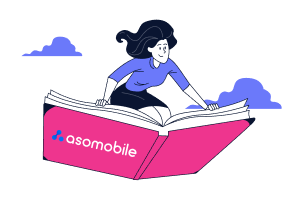
- ASO Basics for Google Play and App Store Apps
- Creating and Analyzing the Semantic Core
- Text Optimization for App Store and Google Play
- Icon, Screenshots, Video. A/B Testing
- Featuring, Similar Apps, Ratings, Working with Reviews
Analytical tools for the formation of semantics
In order to find keywords with all the characteristics and indicators we need, we will turn to mobile application analytics by ASOMobile. There is a very wide range of tools for analyzing not only keywords but also competitors, the mobile application market, download and revenue indicators, and much more. However we will focus on the foundation of optimization - the semantic core.
Even if our app is only at the idea stage, we can already outline the intended functionality, and therefore determine the competitive field. Why is it important? Because we will collect semantics not out of thin air, but based on analytical data.
Incoming information is a list of words that we already have based on logic, functionality, and store tips. The main competitors are similar functionality and, preferably, a high level of installations. We choose our guiding star, and based on it we will form a semantic core.
We will not stray far from the idea of home fitness, but how can we find the very app that will serve as both a base and an example for us? Analytical tools will serve us here, too - the Top Charts in each market and selected country look like this:
In the top 10 of the Health & Fitness category, there was an app that is most similar to ours and has identical functionality, that is, it is our relevant competitor.
The app - JustFit: Lazy Workout & Fit, which takes the third place in popularity among users on the App Store.
The main idea is fitness at home, without additional equipment, sets of exercises and a fitness plan for each user.
We add it to ASOMobile analytics and select the country for which we will collect the semantic core. There are several nuances worth mentioning here.
N.B.
- the semantic core is collected separately for each store (since keywords have different indicators of traffic, popularity, etc.)
- the semantic core is collected separately for each country, for exactly the same reasons. There is even a country that speaks English - and search queries and their parameters will differ in the USA and in the UK).
Now let's return to analytics - we will have several tools as our main field of work:
- App Keywords - here we will see all the keywords by which the selected app is indexed. The more there are, the better, but you shouldn’t forget about their quality either.
- Keyword Monitor - all the key queries that we add to our core will go here. Already at the start there will be the list that we created before working with analytics, and you can also add auto-suggestions from the system there.
- Keyword Finder, Keyword Select, Keyword Suggest, Suggest Checker - a number of tools for selecting keywords.
- Text Analyzer is a text analysis tool that we can use to find keywords in competitor descriptions.
Now let's get started - add a competitor app, our list of requests, use the auto suggestion from analytics:
Already at this stage, we can go to Keyword Monitor and see our semantic core, simply from the search queries that we added and the system’s auto suggestions. Let's pay special attention to the keyword parameters that are now available to us:
Our semantic core for the USA, iOS contains 20 search queries. We will pay special attention to traffic indicators. This is the number of users per day who enter that keyword into an app store search in that country.
As we see from analytics, the search query “just fit” is searched by 1,245 users per day in the United States.
For now, we suggest filling our core with the maximum number of search queries, and then moving on to the analysis process and generating the final list of keywords.
To do this, we will go to the main tool - App Keywords, here there will be all the words for which our competitor has indexing. What we also need, since we are so similar in functionality. Our competitor's indexing is our important benchmark:
We will go through all the search queries and add them to our semantic core; doubts about their relevance can be resolved along the way, or later, when working with the semantic core. How will we check the keyword? Doubts usually arise in several cases; such a request would not have occurred to us, but there are users and the keyword is popular. We also often begin to doubt when a search query is not directly related to our functionality - we simply check it, look at what is happening in the search results and if our competitors are there, then it is worth including it in our semantics. We can check search results directly in analytics:
The search results confirm that this keyword is suitable for us, and in addition, we can see other competitors that interest us and add them directly from the results in the analytics. We can do this with the keys that interest us - add competitors from the search results because they are our source of the most diverse and relevant semantics.
The next step is to use the rest of the keyword search tools. All have different principles of operation but are simple and clear to use:
- Keyword Finder - focuses on the words that we have added to the semantic core and offers other relevant options.
We will add whatever seems suitable and relevant and has traffic. It is necessary to be guided by the latter since words that are of no interest to anyone will not benefit our app. At the same time, including only words with very high traffic in the core will lead to the fact that we will get indexed, but we will end up at the very end of the search results list and users simply will not find us. We maintain a balance and include search queries with different traffic indicators in the core.
- Keyword Select - will require some input information from us. Here, we need to specify the search query that interests us, and the analytics will suggest related keywords.
One by one, we enter the keywords that interest us, such as home workout, fitness for women, fitness plan, and complement our semantic core.
- Keyword Suggest - all app store tips in one place, at the very initial stage of working with semantics, we found out the important role of store tips. Here we can find absolutely all the clues for the keywords that interest us:
All possible key phrases are placed in alphabetical order, so that we can select suitable queries and add them to the semantic core.
Now, we will use the capabilities of the Text Analyzer tool, which conducts a semantic analysis of any text and determines the presence of keywords with all their characteristics.
The first thing we will analyze is the description of our main competitor, which serves as the basis for optimization. The description of this app is already in the analytics automatically, so all that remains is to analyze:
As a result, we receive information about the volume of text, and the number of words used, but most importantly, about the keywords that Text Analyzer detected when scanning this description.
If the keywords suit us and are not yet in our semantic core, feel free to add them.
In addition to the current description, we can analyze the description of any other app. And if necessary, we can manually insert any text that interests us and get a comprehensive analysis.
It's time to return to the result - a draft semantic core with all the added keywords is waiting for us in Keyword Monitor.
Analysis of the semantic core of your app
And so we have formed a very long list of keywords, with traffic, for which our competitors are indexed and they are popular among users of this niche. Now we will follow the principle of relevance - check each search query for compliance with our functionality. Here you should adhere to the following principles:
- Don't be too specific, but don't spread yourself too thin. If our app does not have a calorie counter or nutritional recommendations, we can safely remove these requests. The logic is the following: of course, users may be interested and install our application, but in essence this misleads them. Not everyone who is interested in nutrition facts will be interested in home fitness. And such a non-target setting will lead to deletion, a low rating and, most sadly, a negative review.
- Clearly delineate the boundaries around target queries - for example, a user can search for how to lose weight, and download our home fitness application for this purpose. But if the user is more interested in yoga for calming the mind and mental health, then for our app this will be a somewhat relevant and not at all targeted request. We balance, because sometimes users themselves do not know what they want to find in the market and we are shown using a wide pool of keywords. But we are careful not to mislead the user.
- We evaluate the traffic indicator of the keyword - as mentioned above, we avoid words with zero traffic. No demand - no installations. But if we focus only on high-frequency queries, then this leads to a very low position initially in search results, and very high competition for users. But mid-frequency queries are the most promising, because the competition is not that great, but there are users.
Now let's go through our semantic core - removing everything that is not relevant (meal plans, calorie counters, mental health, meditation and of course other people's brands). Then we will sort everything by traffic indicator; for convenience, you can define all requests into three groups - high-, medium- and low-frequency. Moreover, we also used color labels for each of these groups:
- 🟢 for queries with the highest traffic
- 🟠 for keys with average traffic value
- 🟡 for low traffic search queries
*colors are chosen randomly, and any similarities are random
There is no single value for determining these indicators; the scale will be different for different types of apps, niches and categories. Somewhere 400 requests per day will be in the green zone, and somewhere else it will fall into the yellow zone.
The same is true for the number of keywords in the semantic core. For an app like a calculator, we won’t even get 60 queries. However, for the Photo and Video Editor, it will easily exceed 150 keys.
This is what our semantic core looks like, which consists of 183 key queries - the result of our painstaking work.
We now understand the sources of semantics, analytical tools and types of keywords and can easily form a semantic core for any app or mobile game. But what's next? And then you need to place the existing keywords in the app metadata, according to all the rules and concepts of stores.
It contains detailed and step-by-step instructions for generating app metadata for both Google Play and the App Store. What happens next will not be so labor-intensive, but very important because the semantic core, even the most relevant and carefully verified, will not lead us to the desired goal - indexing.